저는 옴니어스에서 머신러닝 엔지니어 (Machine Learning Engineer) 로 일하고 있는 아드리아 아루팟 (Adrià Arrufat) 이라고 합니다 🙂 제가 옴니어스에 몸 담은지 약 3년 정도가 흘렀는데요, 이 블로그 포스팅에서는 지금까지 일하면서 느꼈던 경험을 바탕으로 옴니어스가 왜 머신러닝 엔지니어로서 일하기 좋은 곳인지에 대해서 공유하려고 해요.
간단한 제 소개에 이어 옴니어스가 어떤 회사인지, 어떤 목표를 가지고 있으며 목표 달성을 위해서 어떻게 일하고 있는지 소개 드릴게요.
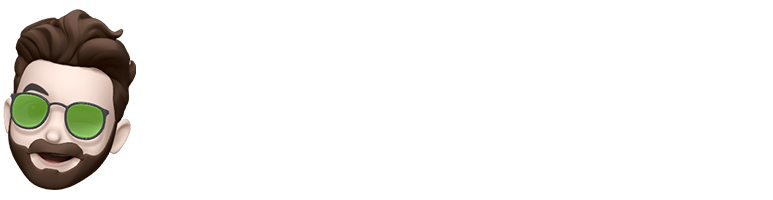
저는 제 고향인 스페인 바르셀로나의 Universitat Politècnica de Catalunya 에서 telecommunications engineering (a.k.a., electrical engineer in US) 으로 학사 학위를 받았어요.
그리고 프랑스의 Orange Labs에서 일하면서 INSA (Institut National des Sciences Appliquées) de Rennes 으로부터 Video compression에 관한 박사 학위를 취득했어요.
박사 취득 이후에는 머신러닝 으로 커리어를 전환하여 Orange Labs에서 2016년 부터 2018년까지 얼굴 인식 기술에 관한 연구를 진행하였습니다.
그리고 2019년 1월 부터 옴니어스에 합류하여 다양한 패션 이미지 인식 관련 연구를 진행하고 있어요.
옴니어스는 어떤 회사일까?
옴니어스는 2015년 8월, KAIST 출신의 머신러닝 전문가들이 설립한 회사입니다. 2015년에는 AI/ML 분야에서 큰 성취점이 있었는데요, 바로 ResNet의 등장입니다. ResNet은 이미지 분류에서 더욱 깊은 신경망 모델을 활용하는데에 성공했으며, 당시 이미지 분류 분야에서 가장 어렵다고 할 수 있는 ImageNet Challenge 에서 당당히 1위를 차지했습니다.
ResNet을 시작으로, 딥러닝 기반 모델들이 수많은 hand-crafted 특징 기반의 모델들을 대체하기 시작했고, 사람의 정확도를 넘어서는 문제들이 점차 늘어나고 있었는데요. 특히 인식과 관련된 문제 (얼굴 인식, 사물 인식, 물체 위치 검출 등) 에서 두각을 나타내고 있었습니다. 이러한 문제들은 사람이 아니면 풀기 어려운 인간의 본성과 관련된 본질적인 문제라고 여겨졌기 때문에 더욱 신선한 충격이었어요 😲
옴니어스는 이러한 AI의 지각 변동을 발판으로 삼아 인간의 본성과 큰 관련이 있는 또 다른 영역인 패션을 첫번째 타겟으로 정했습니다. 과연 사람도 이해하기 어려운 정성적인 패션의 영역을 신경망 기반의 AI 모델이 이해할 수 있을까요?
아래 질문들은 AI에게는 매우 어렵지만 사람이라면 복잡한 생각 없이 쉽게 답변할 수 있는 예시입니다.
- 패션 아이템은 무엇입니까?
- 어떻게 패션 아이템들이 결합되어 하나의 룩을 구성할 수 있을까요?
- 여름 피크닉 스타일의 옷은 어떤 특징들을 가지나요?
- 어떤 패션 속성이 드레스를 웨딩 드레스로 만드나요?
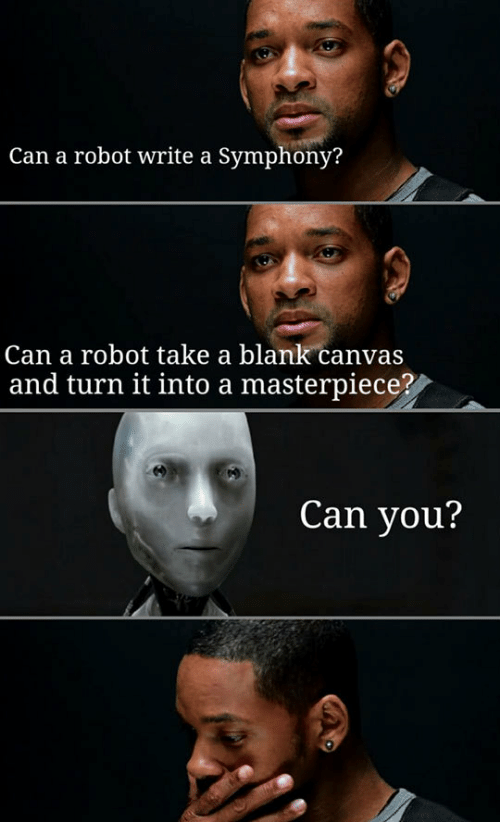
창의성을 수반하는 다른 많은 작업들 처럼 패션을 이해하는 것은 기계에게 매우 도전적인 일이며, 패션을 이해하고 자신만의 방식으로 사용할 수 있는 AI가 있다면 그것은 일종의 예술적인 AI라고 볼 수도 있습니다.
옴니어스가 제공하는 AI 기술을 이해하기 위해 먼저 패션을 이해하는 AI 기술이 달성해야하는 몇 가지 기본적인 문제를 정의하는 것부터 시작 해볼까요?
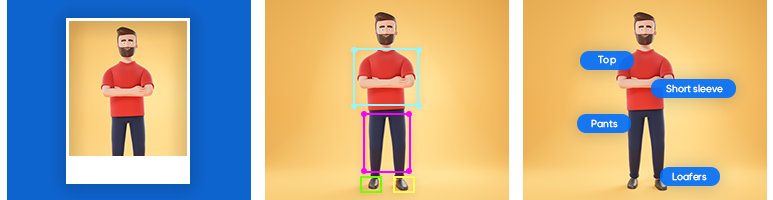
- 이미지에서 패션 아이템(의류, 액세서리, 가방, 보석 등)의 위치 찾기
- 그 패션 아이템들을 더 세분화된 범주로 분류하기
- 각 아이템에 대한 관련 패션 속성 태깅하기 : 패턴, 소재감, 기장, 쉐이프, 핏, 색상, 스타일, ...
누가 이런 기술의 혜택을 가장 많이 받을까요?
한 가지 분명한 답은 패션 소매업자들 입니다. 실시간으로 모든 패션 카탈로그에 관련 속성 태그를 자동으로 달 수 있다고 상상해 보세요. 모든 패션 아이템에는 상세하고 의미 있는 정보가 태깅되어 잠재 고객이 제품을 더 쉽게 발견할 수 있고, 이는 고객의 쇼핑 경험을 크게 향상시킬 수 있습니다.
이것이 바로 옴니어스 태거 (OMNIOUS Tagger) 가 하는 일이며, 옴니어스의 핵심 기술입니다. 옴니어스는 이러한 태깅 기술을 기반으로 유사한 상품을 추천해주는 OMNIOUS Lens 서비스, 패션의 트렌드를 분석하여 맞춤 정보를 제공하는 OMNIOUS Alffy 서비스 등을 통해 다양한 패션 업계의 pain point를 해결해 나가고 있습니다.
머신러닝 엔지니어가 일하기 좋은 환경
다음으로, 왜 옴니어스가 머신러닝 엔지니어로써 일하기 훌륭한 회사인지 얘기해 볼게요 🙂
옴니어스의 가장 가치 있는 자산 중 하나는 AI 모델을 학습하기 위해 지속적으로 발전하는 고품질 & 대용량 AI 학습용 데이터 셋 입니다. 그것이 우리가 패션 분야의 다른 경쟁자들과 차별화되는 점이고, 연구자로서 느끼는 큰 장점 중 하나 인데요. 이러한 데이터 셋 외에도 제가 옴니어스를 가장 마음에 들어하는 부분은:
- 회사의 최종 목표를 향해 나아감에 있어서 각 팀들의 맡고 있는 역할 하나하나가 밀접하게 연관되어 있다는 점과
- 그 결과물이 끊임없이 다른 팀들에게 긍정적인 영향을 미친다는 점입니다.
옴니어스의 AI 연구팀은 패션 아이템 위치 검출기, 패션 속성 인식 모델과 같은 AI 모델들을 개발하고, 더 좋은 성능을 내기 위한 모든 관련 연구를 담당하고 있습니다.
AI 모델의 성능이 좋아지면 좋아질수록, 다음과 같은 회사의 다른 영역의 문제들을 푸는데 도움을 주고 있어요.
- 학습된 AI 모델 기반 자동화된 라벨링 파이프라인 구축
- AI 모델의 신뢰도를 활용한 학습 데이터 검수 속도 개선
- 성능 향상을 통한 제품 경쟁력 강화
하지만 그와 동시에, AI 모델의 성능 개선은 옴니어스의 다른 팀들의 끊임없는 노력으로 부터 만들어진 결과물이기도 해요.
- 패션 전문가로 이루어진 패션 데이터 팀에서는 AI 모델에 학습에 가장 적합한 카테고리를 결정합니다. 이때, 패션 업계의 속성 현황과 더불어 고객의 요구와 기술적 제약 사이에서 최적의 지점을 찾아야 합니다.
- 데이터 플랫폼 개발팀은 옴니어스 내의 모든 데이터가 정확하고 빠르게 흐를 수 있도록 효율적인 데이터 export 파이프라인을 구축하고 누구나 쉽게 사용할 수 있는 데이터 라벨링 환경을 제공합니다.
- 비지니스 팀에서는 새로운 고객을 확보하여 더욱 Challenging한 데이터 및 Use case 제공을 통해 연구팀의 업무를 더욱 흥미롭게 만듭니다.
이런 면에서 옴니어스는 저에게 시간이 지날수록 개선될 수밖에 없는, 스스로 진화하는 self-correction 시스템처럼 느껴집니다.
마무리하며
옴니어스의 미래는 지금부터 시작입니다. 패션 분야에는 여전히 AI로 해결하지 못했던 문제들이 많이 남아 있지만, 이미 수년간 패션 업계의 pain point를 해결해주는 서비스를 통해 축적된 노하우를 바탕으로 패션을 넘어 다양한 도메인으로의 확장을 준비하고 있습니다.
옴니어스와 함께 패션 AI의 새로운 미래를 만들어 가실분들은 언제든지 환영입니다!
Introduction
My name is Adrià Arrufat, and I am a machine learning engineer at OMNIOUS. After over than three years working here, I want to share about why I think OMNIOUS is a great place to work for machine learning engineers.
In this post, after properly introducing myself, we will discuss what OMNIOUS is, its goals, and how does it achieve them.
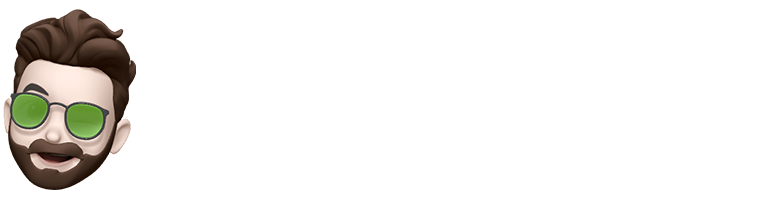
About me
I studied telecommunications engineering (also known as electrical engineering in the US) at Universitat Politècnica de Catalunya in Barcelona, my hometown.
Furthermore, I also hold a PhD in video compression from INSA of Rennes in France, which I obtained while working at Orange Labs.
Thereafter, I changed my career to machine learning at Orange Labs as well. I worked there from 2016 to 2018 on face analysis technologies.
Since 2019 I have been working at OMNIOUS, a company specialized in analyzing fashion items and classifying them into fine-grained attributes.
What is OMNIOUS?
OMNIOUS was founded in 2015 by machine learning experts. At that time, we had experienced a revolution in the machine learning field. To give a little context of what was like around then:
- Neural networks were taking over one of the most challenging competitions in image classification, ImageNet.
- ResNets were the new cool kid in the block: for the first time, increasing the depth of neural networks did not decrease the accuracy.
As a result, neural networks were replacing other models with hand-crafted features, most notably in face detection and recognition. These tasks are inherently related to our human nature, so it was mind-blowing to see deep neural networks outperform human accuracy.
OMNIOUS decided to tackle another task very related to the human nature: fashion. Could these neural networks understand what fashion is? Understanding fashion is hard, do we even understand it?
These are some tasks that we do every day without thinking, but are very challenging for a machine:
- What do we mean by a fashion item?
- How fashion items can be combined to form an outfit?
- What do we mean by summer-looking clothes?
- What makes a dress a party dress?
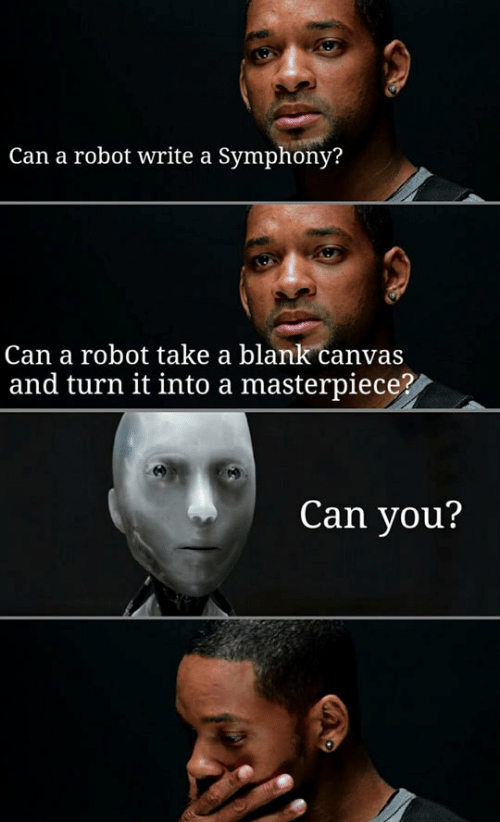
Like many other tasks that involve creativity, fashion is very challenging for machines, and we could even argue that a machine that could understand fashion and use it in its own way would look like an artistic AI.
Let's start by defining some basic goals that a technology that understands fashion should be able to accomplish:
- Finding fashion items (clothing, accessories, bags, jewelry, etc.) in images
- Classifying those fashion items into fine-grained categories
- Tagging the relevant attributes for each item: print, material, length, shape, fit, color, style…
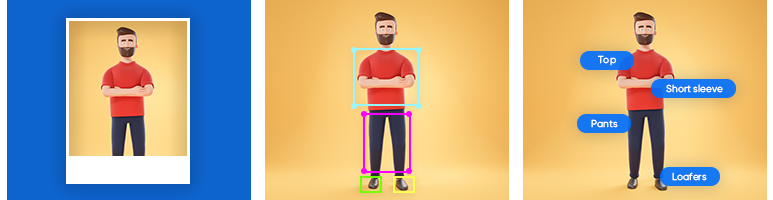
We can now build on top those tasks to create higher level and more semantically meaningful applications:
- Finding similar fashion items according to several criteria
- Combining different fashion items according to a particular style
- Fashion trend analysis on social media
Who would benefit the most from such a technology?
The obvious answer is fashion retailers: imagine if you could automatically tag all your fashion catalog instantly.
All the items would have really detailed and meaningful labels, which would make your products more discoverable by potential customers.
That is, in fact, what the OMNIOUS Tagger does, the core technology of OMNIOUS.
The OMNIOUS Tagger uses high performant detectors and classifiers, trained on a custom internal dataset, carefully curated by fashion experts.
The loop
Now that we understand what OMNIOUS does, I will introduce what I think it makes is an excellent place to work for machine learning engineers.
One of our most valuable assets is the high-quality, ever-evolving dataset we created from scratch to train our models. That's what sets us apart from other actors in the field.
However, what I like most about OMNIOUS is how every team is essential to the end goal of the company, and their results make an impact from which every other team benefits directly.
From my perspective: the machine learning team at OMNIOUS is in charge of developing dependable and high-quality models: detectors, classifiers, semantic segmentation, etc.
The better these models perform, the more these tasks improve:
- Automated labelling pipeline for new images
- Easier job for the reviewers of the automatically labeled data
- Selling the product to our customers
However, at the same time, our task is only possible because other teams put a great deal of effort into their jobs:
- Fashion experts and product managers decide which categories make sense in our models: we need to find the sweet spot between customer needs and technical constraints
- Developers create an easy-to-use framework for a smooth labelling and data exporting experience
- Business team gets us new clients, with more challenging datasets and use cases, which make our job even more interesting.
OMNIOUS feels like a self-correcting system that is bound to improve as time goes by.
The Future
OMNIOUS is just getting started. The fashion domain is far from being a solved problem for AI. However, we are already working on how to expand all the knowledge we acquired over these past few years and apply it to other domains.
Join us to shape the future of AI, exciting times ahead!